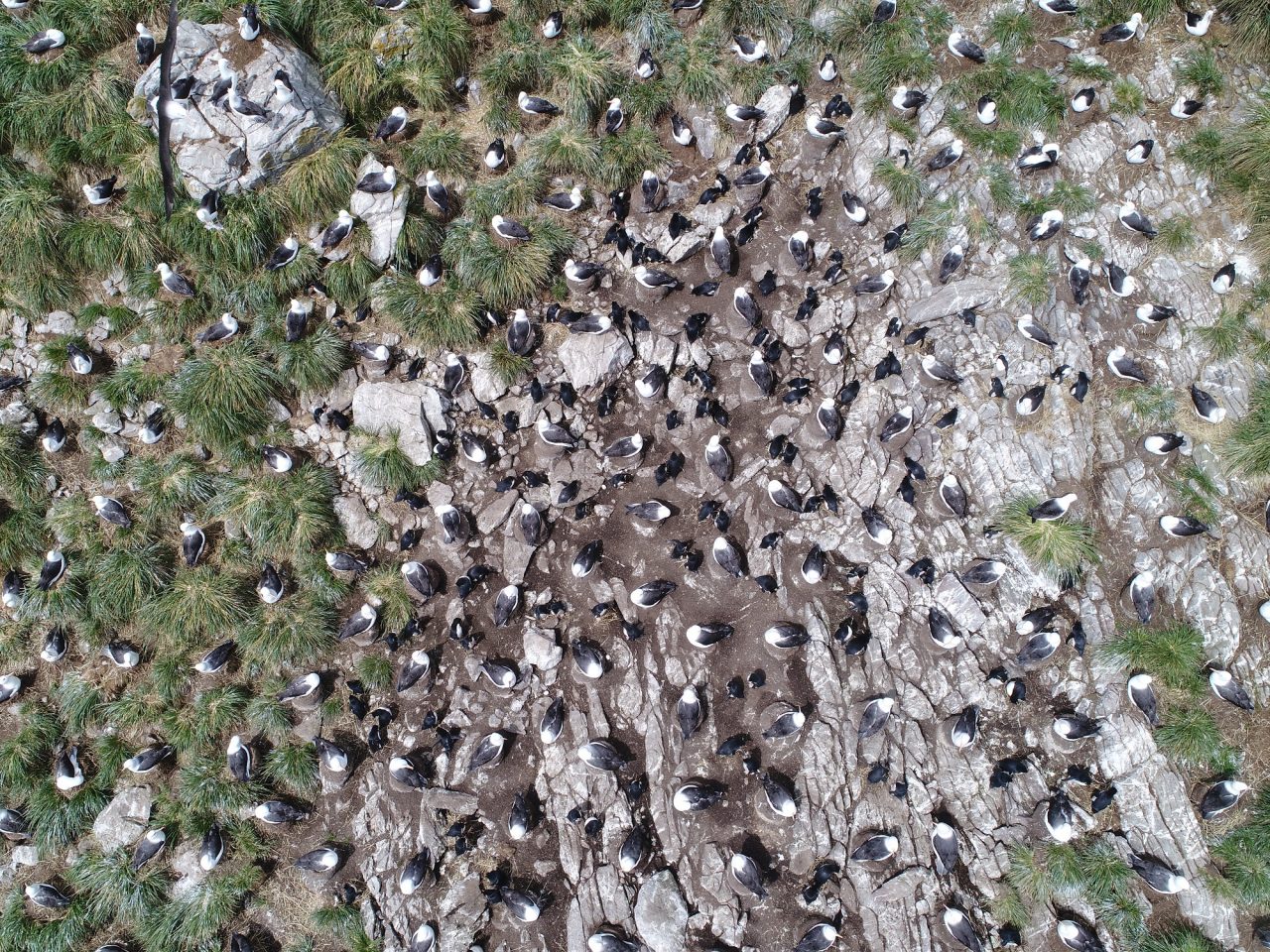
Just picture it.
You, standing at the top of a steep slope blanketed by seabirds.
Sponsor Spotlight
The seemingly endless mass of black-browed albatrosses and penguins create a patchwork quilt of white, brown and black with pops of orange leading down to the choppy blue waters of the Atlantic.
You traveled by air and sea to hike the rugged terrain in this particular spot in the Falkland Islands, this place cluttered by the noise of the albatrosses’ calls.
You made it. Now, start counting.
This boots-on-the-ground method has for decades been the traditional way in which wildlife biologists count seabird colonies, large and small.
Sponsor Spotlight
But the use of drones paired with emerging software technology is starting to change that, affording biologists a means of more quickly and accurately monitoring large seabird populations, a job researchers point out is important because these populations help, among other things, detect changes in the environment.
“Being able to very quickly and easily get that perspective from above really changes the situation,” said Dr. David Johnston. “At that point in time you see every single bird that’s there and there are a lot of places where you just can’t do that.”
Johnston, associate professor of the practice of marine conservation ecology at the Duke University Marine Lab in Beaufort and director of the Duke Marine Robotics and Remote Sensing Lab, took part in a recently published study that is one of the first to successfully use “deep learning” as a way to count large seabird colonies through drone imagery.
Duke researchers partnered with the Wildlife Conservation Society to collect drone imagery of large colonies of black-browed albatrosses and southern rock upper penguins on the Jason Islands, a small island chain off the Falkland Islands in the south Atlantic Ocean.
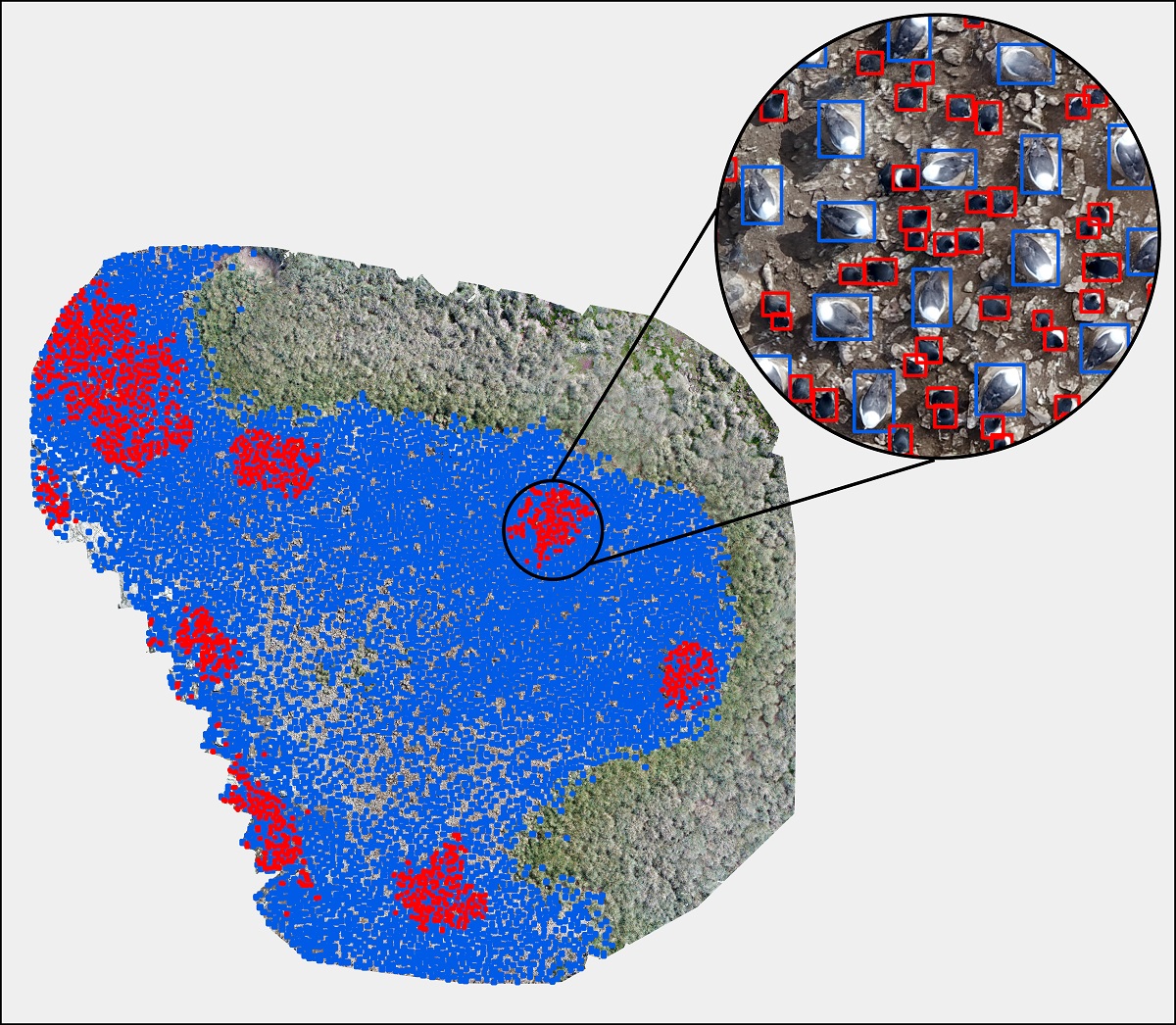
Steeple Jason Island. Graphic: Madeline Hayes, Duke Marine Robotics and Remote Sensing Lab
The Falklands hold about 70% of the world population of black-browed albatross.
Images were collected within a span of few days in 2018 and again in 2019.
Those images were strung together to create a map and count of the colonies through new and emerging software.
“The drones are small. They’re very affordable. They’re easy to use and a researcher on the ground with a small team or even by themselves could actually do these surveys,” Johnston said. “The problem is that you have thousands and thousands and thousands of photos that you have to deal with and it turns into a big data problem really quickly and that’s where the software side of things come in.”
In all, about 10,000 drone images were handed over to Madeline Hayes, a research technician in the lab, whose job it was to create the models that identified the birds.
The deep learning model is like this: the neural network system gives the computer examples of what species needs to be identified. The computer looks at those images and finds the unique properties like colors, edges and dimensions. This information is distilled down into a set of mathematical equations that can be used to apply to new images.
“The most time intensive part is labeling the birds,” Hayes said. “When that was all said and done, that probably took me 30 to 40 hours.”
The work, she said, was challenging at times, particularly since this was her first experience with deep learning. She has a background working with drones, but, until this research, had not stepped over to the world of artificial intelligence.
The next part of the process was hands-off as the models Hayes created to pinpoint each species trained the software which birds to identify.
Once the software was able to identify each species, it looked at the maps Hayes thread together using the drone imagery — a dozen maps in all — and counted the birds.
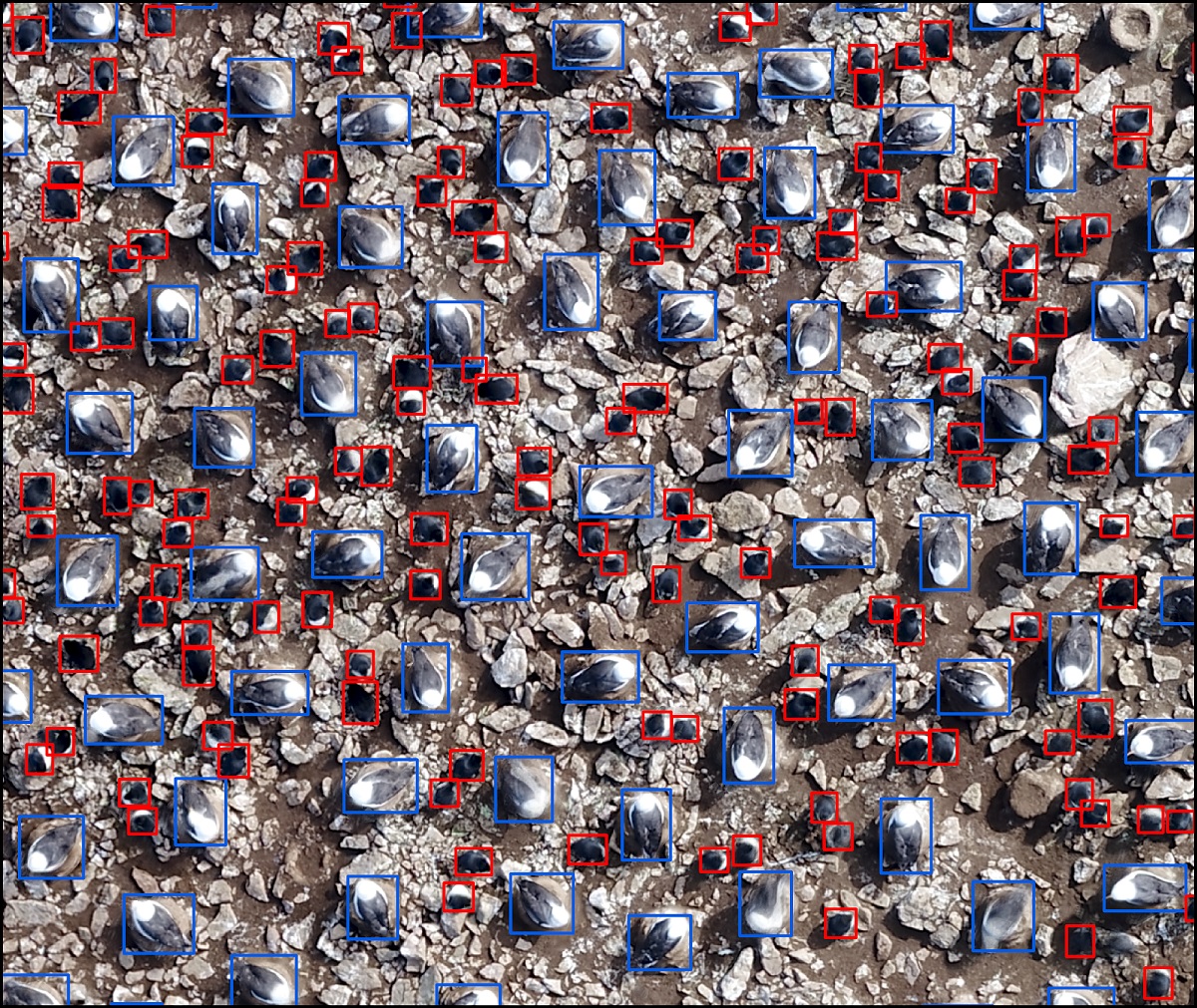
rockhopper penguin detections in red. Graphic: Madeline Hayes, Duke Marine Robotics and Remote Sensing Lab
Within a couple of hours, the software counted about 100,000 albatrosses at one site. Within about 20 minutes, the software counted up to 10,000 penguins at another site.
The overall results: the model for the black-browed albatross identified that species at an accuracy of 97.66% and the model for the southern rockhopper penguin achieved an accuracy of a little more than 87%.
The technology allows researchers to generate more data and gain a better understanding of seabird populations in a much shorter amount of time than the traditional counting methods.
“Seabirds are very sensitive to changes in the environment and they are also subject to all sorts of other things like bycatch in fisheries and so trying to detect changes in their population sizes is very challenging, but it’s a key component for understanding how well they’re doing,” Johnston said. “If the population starts to decline, then we have an understanding something’s either going on in terms of their interactions with people or maybe there’s a change in the environment that’s causing their populations to decline. The more data that you have to be able to do that the more likely you’re able to detect that change before it becomes a real problem.”
These same tools may be used to count an array of large animal colonies, including sea lions.
Here in North Carolina, drones have been used to count federally-threatened wood storks at Warwick Mill Bay, one of the state’s few remaining large, intact Carolina Bays, near Lumberton.
Wood storks nest in trees, which makes it challenging to count them from the ground, said Lindsay Addison, a coastal biologist with Audubon North Carolina.
“From the ground you get a certain view of the colony and from above you get a certain view of the colony,” she said.
Every three years, Audubon counts colonies on the ground per a statewide census. Audubon has not used drones to count shorebirds, Addison said.
“It’s a very situational thing in the sites that we have,” she said. “There’s so many different variables, but having more tools in the tool box would be good.”
The computer code Duke researchers put together is available to the public to download.
“We really wanted to illustrate that ecologists, biologists, wildlife biologists and so forth could build these deep learning models that could really help them in monitoring wildlife and looking at population dynamics,” Hayes said. “Those professionals often aren’t coming from a computer science background. We’re just going to keep going up and up and up with this type of work. I envision the future as a fully automated pipeline.”